SlaBins: Fisheye Depth Estimation using Slanted Bins on the Road Environments
ICCV 2023
Abstract
Although 3D perception for autonomous vehicles has focused on frontal-view information, more than half of fatal accidents occur due to side impacts in practice (e.g., T-bone crash). Motivated by this fact, we investigate the problem of side-view depth estimation, especially for monocular fisheye cameras, which provide wide FoV information. However, since fisheye cameras head road areas, it observes road areas mostly and results in severe distortion on object areas, such as vehicles or pedestrians. To alleviate these issues, we propose a new fisheye depth estimation network, SlaBins, that infers an accurate and dense depth map based on a geometric property of road environments; most objects are standing (i.e., orthogonal) on the road environments. Concretely, we introduce a slanted multi-cylindrical image (MCI) representation, which allows us to describe a distance as a radius to a cylindrical layer orthogonal to the ground regardless of the camera viewing direction. Based on the slanted MCI, we estimate a set of adaptive bins and a per-pixel probability map for depth estimation. Then by combining it with the estimated slanted angle of viewing direction, we directly infer a dense and accurate depth map for fisheye cameras. Experiments demonstrate that SlaBins outperforms the state-of-the-art methods in both qualitative and quantitative evaluation on the SynWoodScape and KITTI-360 depth datasets.
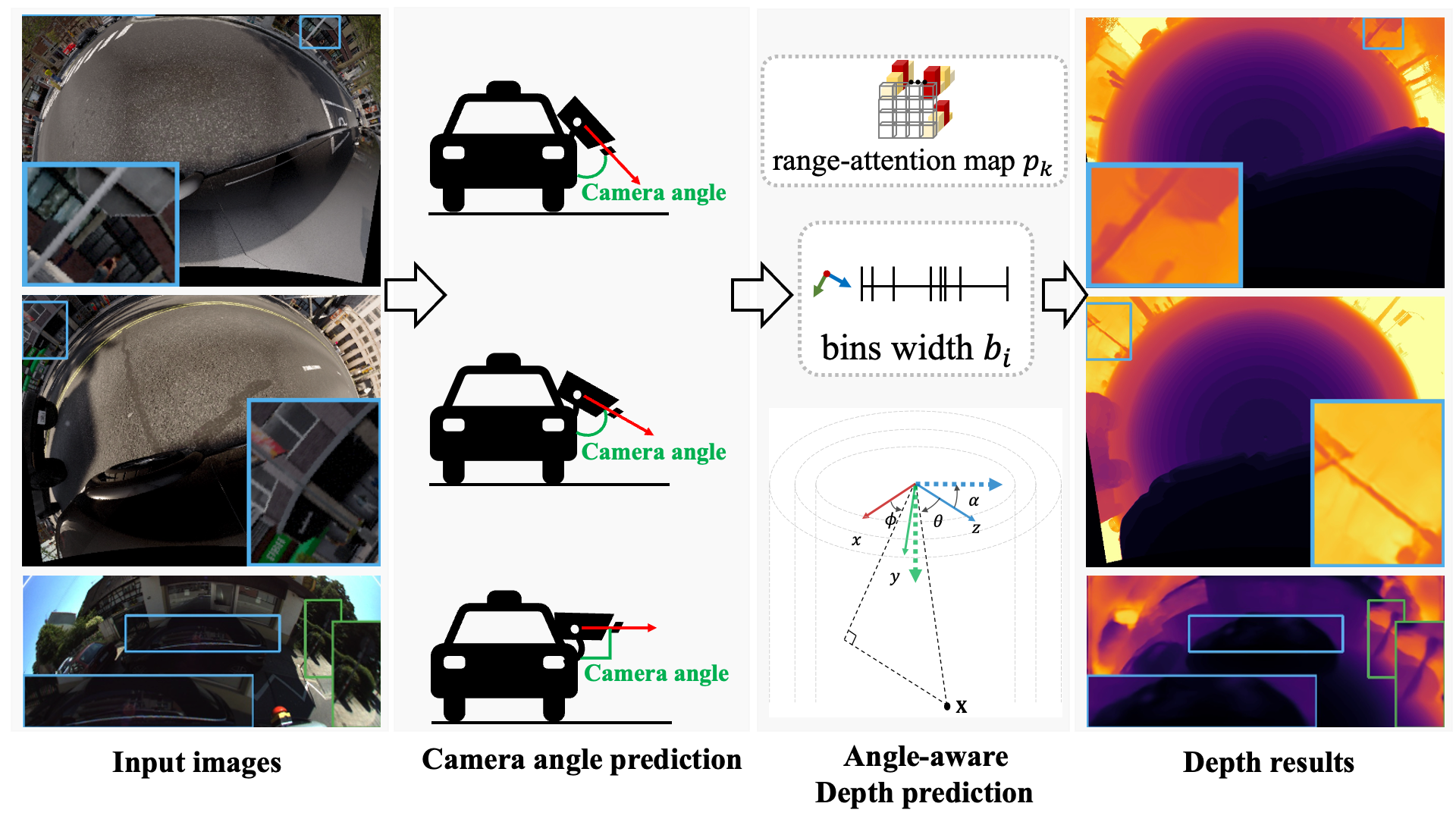
Methodology
Inspired by the geometric property of the road environments, we propose a new fisheye depth estimation framework. Specifically, we introduce a slanted MCI representation, which allows us to describe a multi-layer cylindrical image orthogonal to the road ground regardless of the viewing direction of the camera. Based on the slanted MCI representation, our SlaBins module estimates the adaptive bin widths and per-pixel probability map in the orthogonal coordinate, which provides depth information invariant to the camera viewing direction. We then combine the estimated depth information with the slanted angle estimated by the slanted angle prediction module. Through this process, we can directly estimate a dense depth map for fisheye cameras.
Depth Estimation Results
Estimated depth results on SynWoodScape and KITTI-360 dataset.
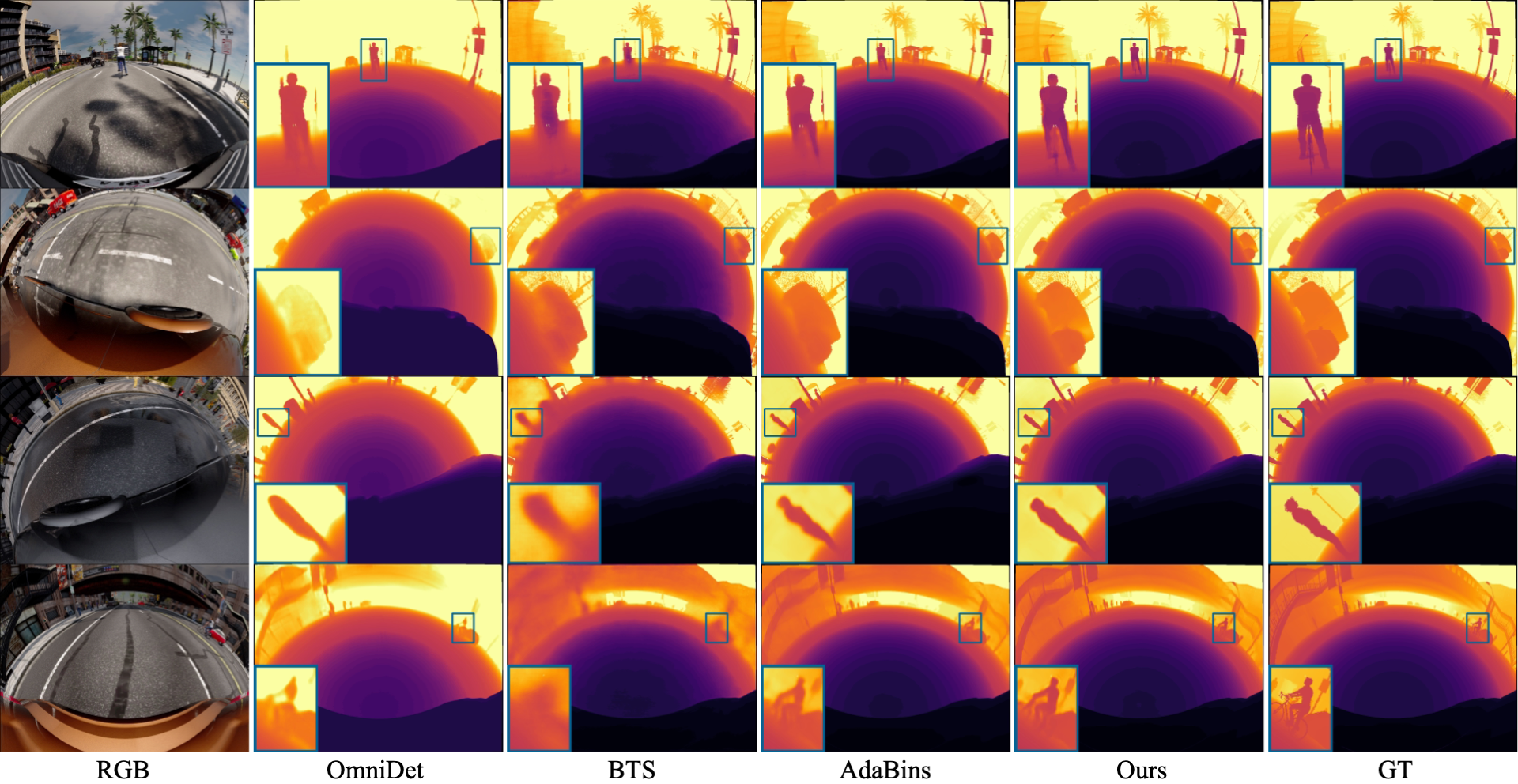
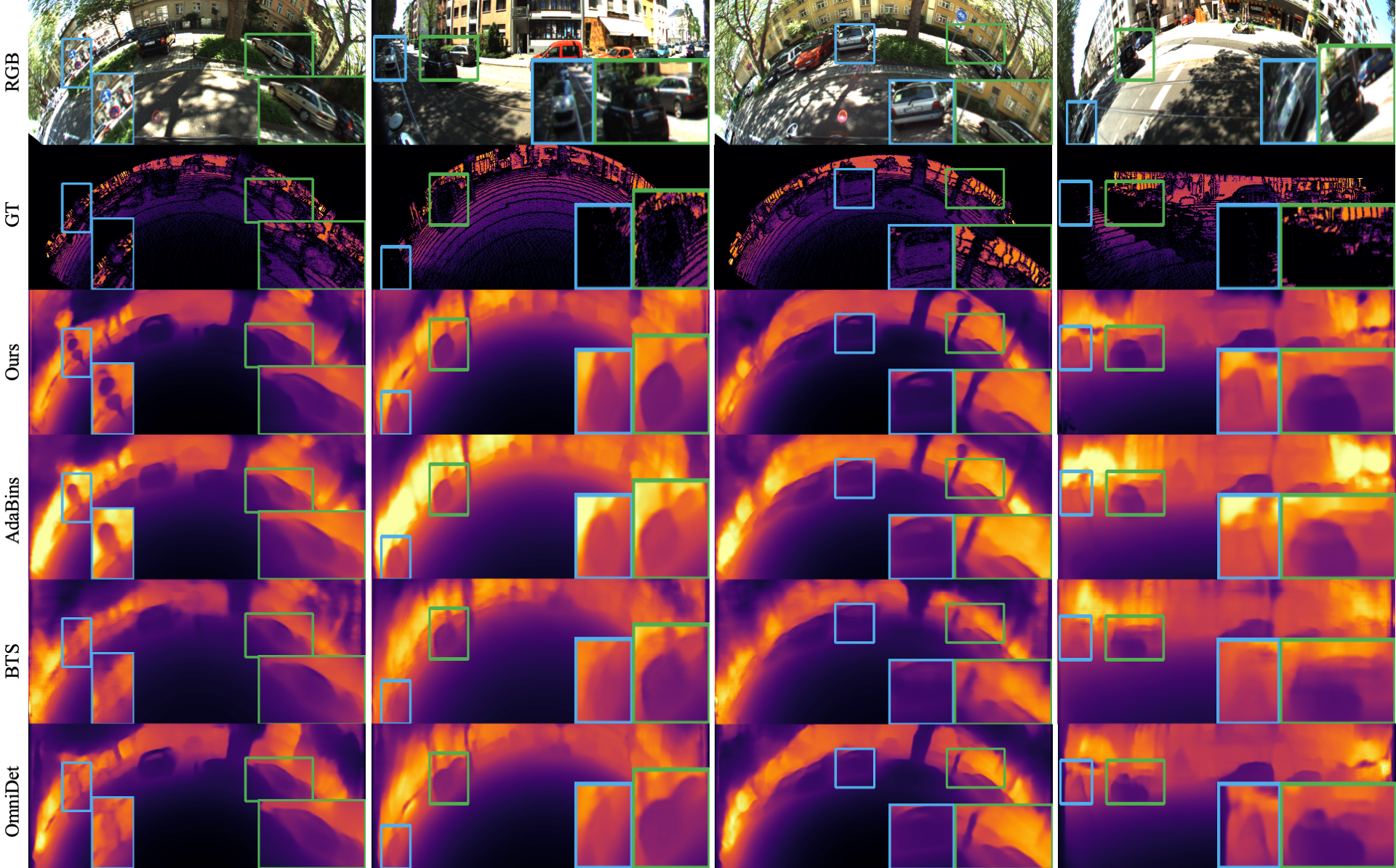
Ablation studies
To verify our slanted MCI representation, we did an ablation study with Original MCI. The visualization results are the predicted depth results and depth clustering results on XZ distance in the orthogonal coordinate.
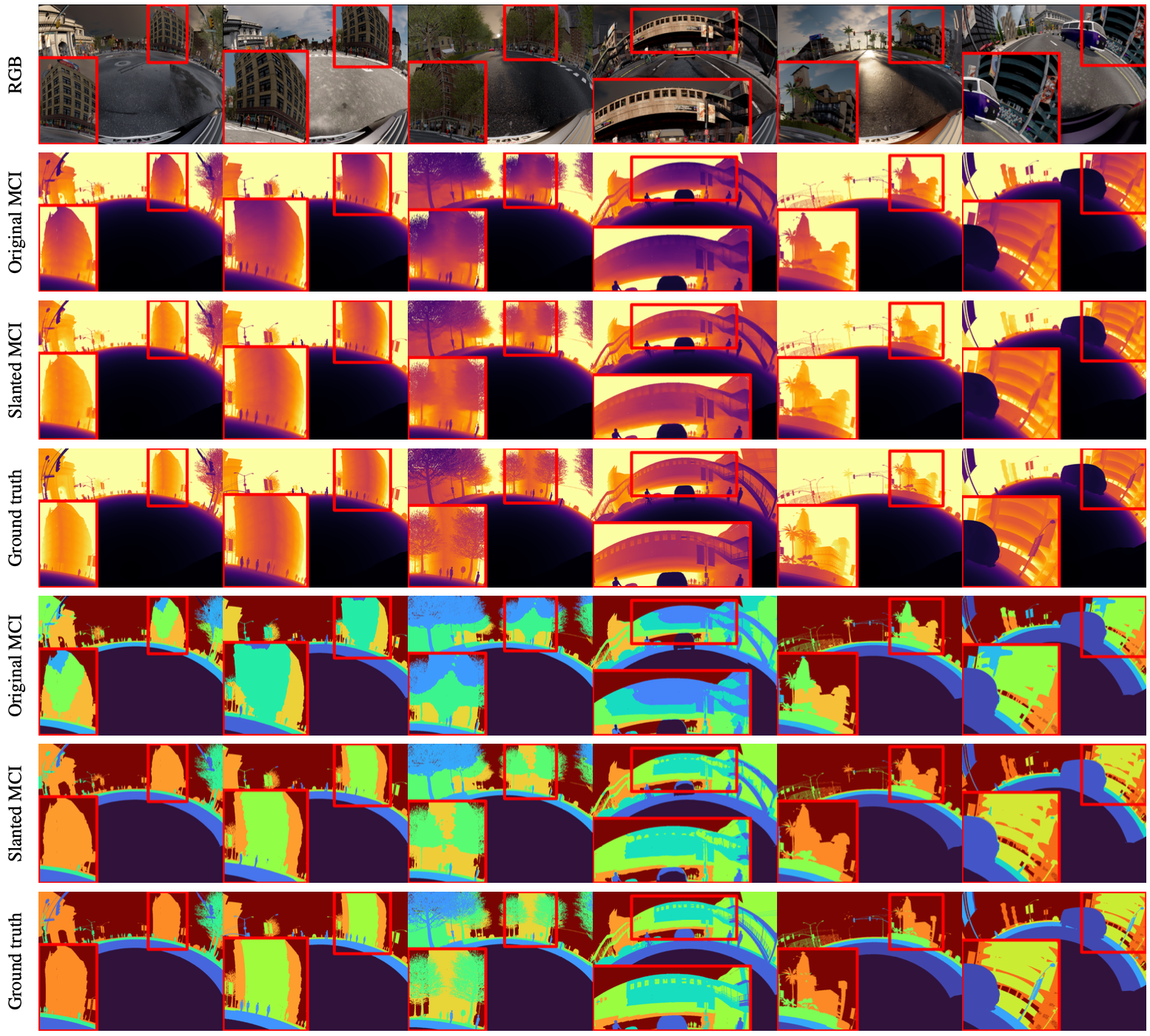
Acknowledgements
This work was supported by 42dot Inc., Institute of Information & communications Technology Planning & Evaluation (IITP) grant funded by the Korea government (MSIT) (No.2020-0-01336, Artificial Intelligence Graduate School Program (UNIST)) and the National Research Foundation of Korea (NRF) grant funded by the Korea government (MSIT) (No. NRF-2021R1C1C1005723).
BibTex
@InProceedings{Lee_2023_ICCV,
author = {Lee, Jongsung and Cho, Gyeongsu and Park, Jeongin and Kim, Kyongjun and Lee, Seongoh and Kim, Jung-Hee and Jeong, Seong-Gyun and Joo, Kyungdon},
title = {SlaBins: Fisheye Depth Estimation using Slanted Bins on Road Environments},
booktitle = {Proceedings of the IEEE/CVF International Conference on Computer Vision (ICCV)},
month = {October},
year = {2023},
pages = {8765-8774}
}